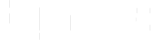
As AI Regulations and Price-Fixing Allegations Pick Up, New Research on Algorithmic Collusion Offers Insights for Executives and Attorneys
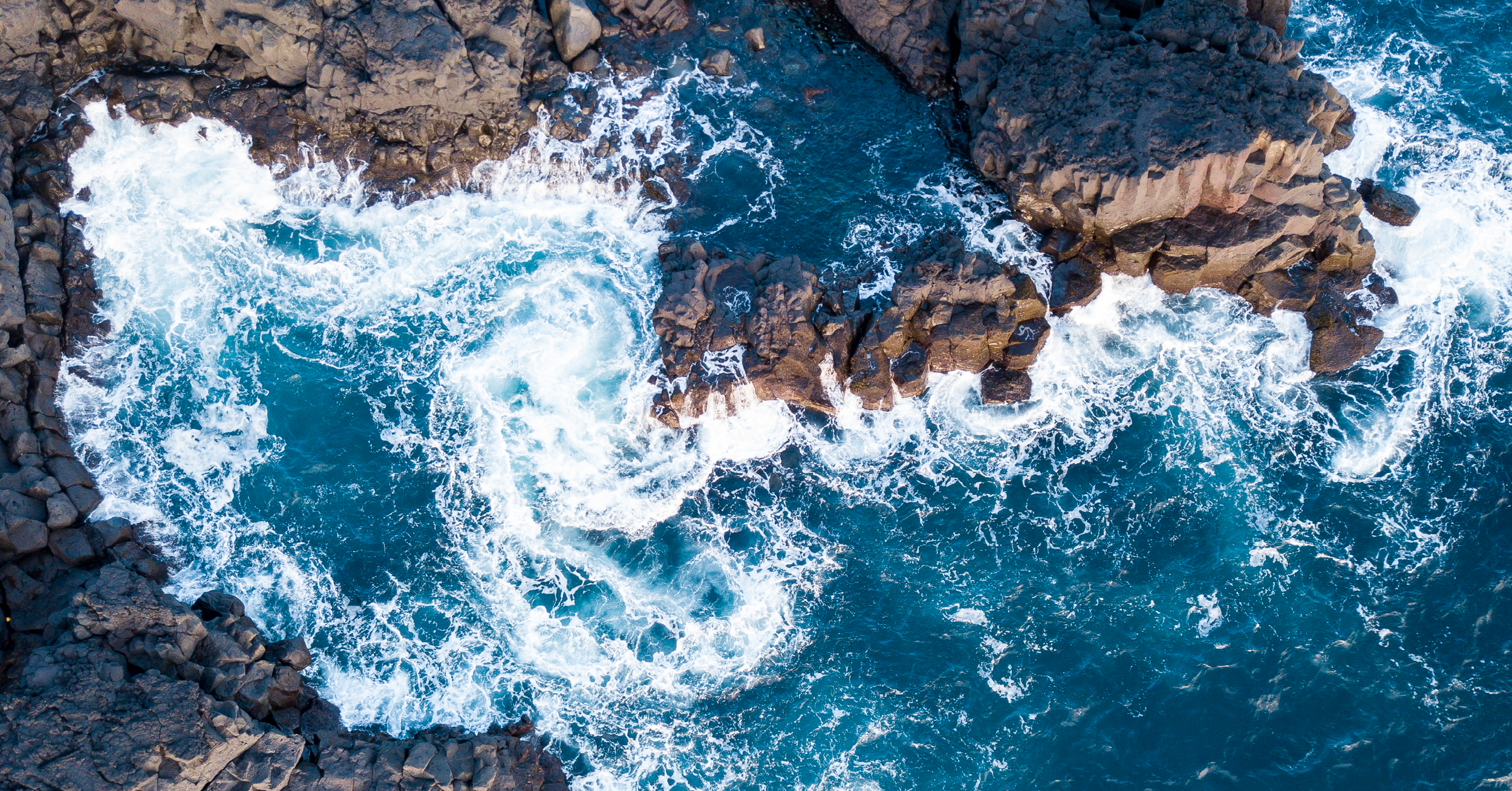
In the first of a two-part series, Ai Deng discusses how algorithms affect prices, the plausibility of algorithmic collusion, and the role of algorithmic design in determining whether a pricing algorithm sets supracompetitive prices
A wave of new price-fixing allegations and heightened regulatory scrutiny of artificial intelligence (AI) tools are putting businesses that use pricing algorithms at risk—underscoring the need to better understand the implications of algorithmic pricing on market competition.
The past two years have seen several civil antitrust complaints against algorithmic pricing providers and their users in the US, as well as warnings from the Federal Trade Commission, the UK’s Competition and Markets Authority, and other antitrust authorities. Lawmakers in the US even proposed legislation aimed at preventing the use of pricing algorithms by companies to fix prices.
Recent academic research offers insights into the inner workings of pricing algorithms, supporting the call for a more nuanced approach to the possibility of algorithmic collusion.
The first of this two-part series focuses on three main topics: how algorithms affect prices, the plausibility of algorithmic collusion, and the role of algorithmic design. The second part will focus on the implications of using third-party pricing algorithms.
Defining Algorithmic Collusion
Collusion, traditionally defined as “a meeting of the minds,” becomes less clear when applied to algorithms, whose key components are compilations of computer codes.
Harrington (2018) provides a definition rooted in the economics of collusion: “collusion is when firms use strategies that embody a reward-punishment scheme that rewards a firm for abiding by the supracompetitive outcome and punishes it for departing from it,” so “a ‘collusive strategy’ refers to a reward-punishment scheme.” This definition has been adopted in most recent studies of algorithmic collusion.
To the extent that the use of pricing algorithms leads to higher prices in certain situations, analyzing algorithmic pricing behavior—rather than simply examining prices—is crucial in determining whether algorithmic collusion exists.
How Pricing Algorithms Affect Prices
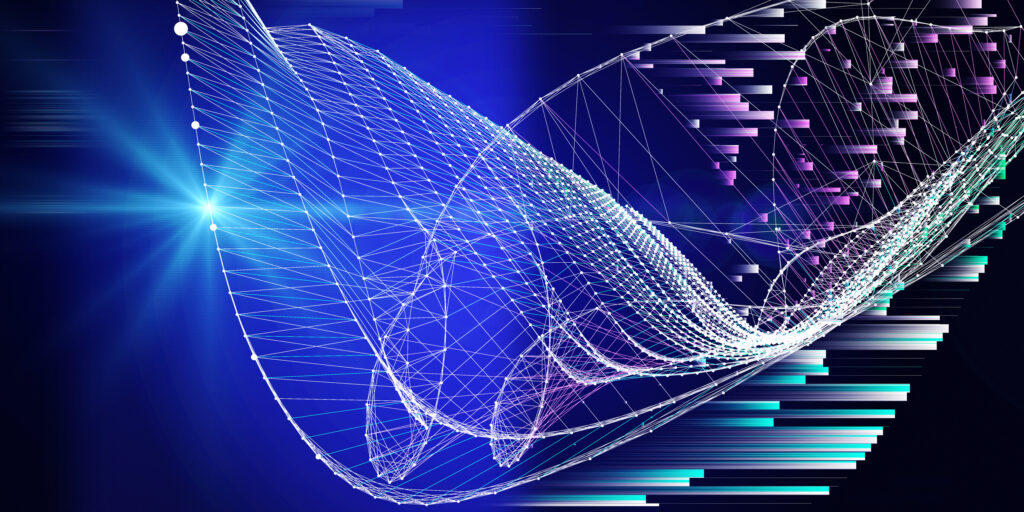
Though not all mechanisms are collusive based on the definition above, economic literature has revealed subtle ways in which pricing algorithms—sometimes with human intervention—can impact prices.
For example, Hansen et al. (2021) demonstrated in a theoretical model that, under some conditions in an oligopolistic market, firms behaving as monopolists and using certain algorithms to experiment with prices can lead to supracompetitive prices (see Cooper et al. (2015) for a similar result). In these instances, the algorithms intuitively set higher prices because the firms mistakenly underestimate the demand responses to such prices. Though high prices may be the ultimate result, these studies show that these prices are not necessarily a result of reward-punishment schemes.
Relatedly, Brown and MacKay (2023) found that different firms’ pricing algorithms choosing and committing to different pricing frequencies (i.e., how often the prices would be updated) can lead to higher prices, even without explicit communications or collusive strategies such as a reward and punishment scheme. For this reason, the authors argue that algorithms can fundamentally change how firms compete by potentially enabling them to increase prices and profits without resorting to collusion, therefore reducing the incentive for collusion in the first place.
Rethinking Autonomous Algorithmic Collusion
On the other hand, Calvano et al. (2020) presented an experimental study suggesting that a certain algorithm—the Q-learning algorithm—could autonomously learn to adopt reward-punishment schemes without explicit communication. While there is no evidence that such an algorithm is in fact used in the real world, their findings were viewed as strong evidence for the plausibility of autonomous algorithmic collusion (see Deng (2019) for a more in-depth discussion).
However, recent studies have questioned these findings. For example, Epivent and Lambin (2024) investigated Q-learning algorithms’ responses to price increases, expecting a reciprocal response if the algorithms were truly collusive. Instead, they observed price wars following both price cuts and increases, casting doubt on whether the algorithms genuinely collude or simply fail to compete effectively.
Similarly, Abada and Lambin (2023) coined the term “seeming collusion” to describe the outcome reached by their algorithms, which do not raise prices to the monopoly level and also appear to punish both “pro-collusive” and “pro-competitive” deviations. The researchers suggest that observed reward-punishment schemes may stem from imperfections in the algorithmic learning process rather than algorithmic sophistication, implying that more advanced algorithms may alleviate concerns about higher prices set by these machines.
Den Boer et al. (2022) further examined Calvano et al.’s findings and argued, among other things, that if a competitor adopts the Q-learning algorithm, it can be exploited by other algorithms that would disadvantage the adopter. The study concludes that simulations presented by Calvano et al. do not provide sufficient evidence that Q-learning algorithms systematically learn collusive strategies.
The Significance of Algorithmic Design
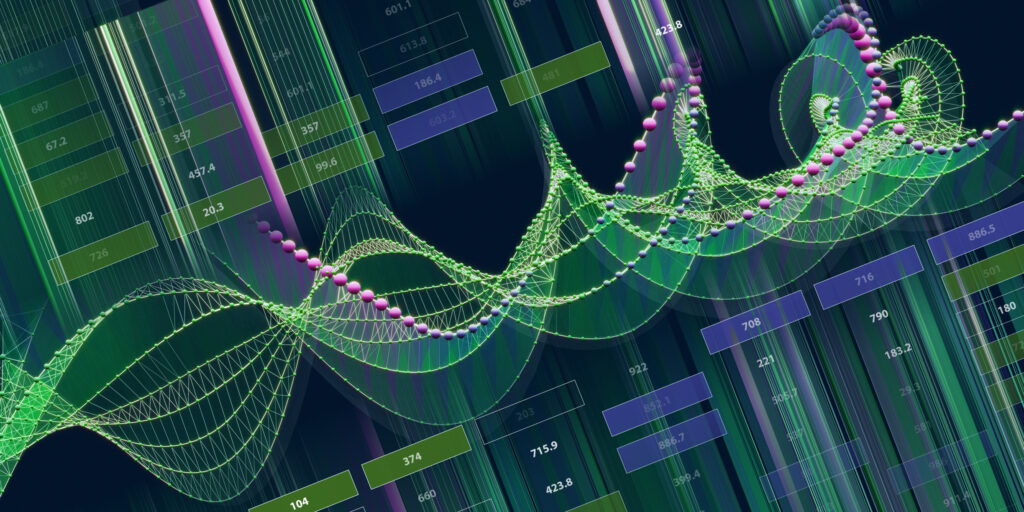
When viewed in retrospect, it is perhaps unsurprising that an algorithm’s design plays a significant role in its pricing behavior. Asker et al. (2024) demonstrated that Q-learning algorithms can set supracompetitive prices even without the features of a collusive strategy. They also showed that when the algorithm takes into account basic economic principles, such as downward-sloping demand, prices can be significantly lower (see Banchio and Skrzypacz (2022) for a similar finding in an auction context).
Importantly, research has shown that an algorithm can indeed be explicitly designed to collude. For example, Meylahn and den Boer (2022) developed a pricing algorithm that can collude when profitable for both firms in a duopoly, but prices competitively otherwise (see also, Loots and den Boer (2023) for their “collude-or-compete” algorithm, which switches between collusive and competitive modules).
Another study, published in 2023, introduced the concept of “adversarial collusion,” where one firm’s algorithm manipulates competitors’ algorithms into collusion. While the researchers argue that deploying such an algorithm may not violate antitrust laws—as there is no explicit coordination or agreement between competitors—antitrust agencies will likely scrutinize firms that knowingly deploy pricing algorithms explicitly designed to collude.
Conclusion
Recent research has deepened our understanding of the complex ways in which pricing algorithms can affect prices. Some studies suggest that algorithms can increase prices without reward-punishment schemes. Others have identified non-collusive reasons for the supracompetitive prices set by simple profit-maximizing algorithms.
At the same time, the efficiency that algorithms provide to companies can be easily recognized. For example, an algorithm may enable a firm to respond more rapidly to changes in its costs and demand and adjust pricing accordingly. Given these and other procompetitive benefits, restrictions imposed by regulators on algorithmic design or input should be carefully vetted.
While it is possible to explicitly design collusive algorithms, the algorithmic design process can be uncovered by antitrust enforcers and parties in litigation via fact discovery. Therefore, it is prudent for companies adopting a pricing algorithm to understand its inputs and functions, and how it increases profitability or helps achieve other business objectives.
… it is prudent for companies adopting a pricing algorithm to understand its inputs and functions, and how it increases profitability or helps achieve other business objectives.
Third-party pricing algorithms have also attracted much attention following recent litigation against hotel chains and multifamily rental companies. The next part of this series will focus on the use of these algorithms and discuss how they may affect prices and market competition.